Relying on legacy governance systems and manual methods to monitor data may expose organizations to significant security, reputational and regulatory risks.
Considering that data governance is an obligation that businesses must now abide by, does it follow that businesses must now implement Machine Learning (ML) Models and/or automation for a policy-driven approach to data governance?
Can one exist without the other?
To gather some insights on the value of incorporating machine learning into data governance CybersecAsia.net interviewed Calvin Hoon, Regional VP (Asia), Rubrik.
CybersecAsia: What are the benefits of a data governance approach using machine learning?
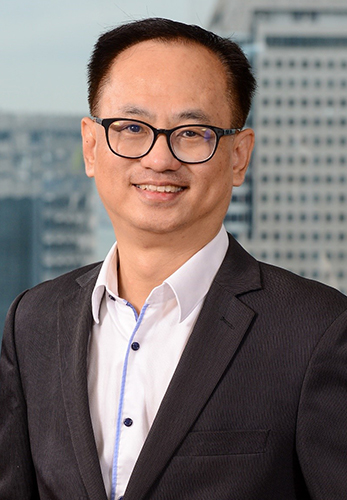
Calvin Hoon (CH): As the volume of enterprise data grows, governance approaches relying on manual oversight alone have the ability to create potential visibility gaps, posing a serious IT risk.
For example, the recent breach of Singtel’s Australian subsidiary Optus exposed personally identifiable information, posing serious risks to customers and the company alike. When firms are implementing new technology or making updates to current systems, ML can be used to lend a ‘second set of eyes’ to help minimize vulnerabilities and help ensure ‘policy-driven’ data governance is followed. This is an approach where IT administrators utilizing ML are provided with a consistently monitored control center from where they can easily manage and support data governance policies.
This control center with ML models can automatically identify sensitive data types (any information that could jeopardize an organization’s competitive advantage or incur legal/risk repercussions if stolen), locations, and usage across platforms to help enforce preset protection parameters (policies).
Today, ML is a crucial part of data security for IT leaders as it provides real-time clarity into the location, access level, and security controls of sensitive business data and helps ensure sensitive data remains compliant with regulatory frameworks.
CybersecAsia: Would effective data governance reduce all-around costs, or is the cost-savings to be derived from being protected from cyber threats, or both?
CH: While overall data architecture cost is largely managed by the IT department, other departments’ shadow IT spending on various third-party business applications can often go unaccounted for within the “IT spend” bucket.
Identifying and connecting these scattered data silos can cost valuable time, and in many cases lead to security oversights. IT and security teams must work together so organizations are making the right investments to protect their data and ensure that departments across the company are compliant with local regulations and data governance mandates.
A unified and scalable solution provides IT teams with a platform to secure and identify organization-wide data requirements, manage the attack surface, and ensure necessary security measures. This can lead to savings from reduced operational costs as well as increased insulation from cyber threats.
CybersecAsia: What should APAC businesses consider in regard to incorporating a policy-driven approach to data governance?
CH: While it may be tempting for firms to keep the status quo and continue with disparate legacy governance systems, many of those solutions are not optimal for use in keeping track of the large amounts of sensitive data that organizations have access to.
As data continues to expand and become increasingly fragmented across enterprise/SaaS/cloud, it is of the utmost importance that businesses prioritize a policy-driven approach to data governance.
Ransomware is one of the biggest enterprise risks today and the likelihood of an attack happening to any business is no longer theoretical, it is simply a matter of when. Relying on legacy governance systems and manual methods to monitor data may expose organizations to significant security risks, reputational damage, and regulatory violations.
Machine Learning models empower IT departments and organizations as a whole with a simple, cost-effective and scalable solution to centralized data governance. They also fortify security measures to offer better protection and response to ever-evolving cyber threats.
CybersecAsia thanks Calvin for his insights on trends in employing machine learning in data governance.