Just as AI can relieve IT defenders of menial threat vigilance tasks, hackers can poison that AI’s training data insidiously.
With the rise of AI and ML adoption, one emerging threat is set to unleashed on industries that are not cognizant of the weak links in smart data processing and analytics.
Threat actors are now looking at messing with data in ways that can be used for cyberattacks. For example, they can do a lot just by changing data for a recommendation engine. From there, they can get someone to download a malware app or click on an infected link. This kind of “data poisoning” involves interfering with a machine learning model’s training data.
According to Palo Alto Networks Field Chief Security Officer (JAPAC), Ian Lim, when attackers tamper with data used to train AI models or detect cyber threats in the network, all kinds of subtle and severe errors can creep into the business, possibly resulting in untold levels of social and legal consequences. CybersecAsia.net interviewed him to find out more…
CybersecAsia: How are threat actors using data poisoning to infiltrate the very tools defenders are using to spot threats?
Ian Lim (IL): Threat actors can infiltrate a system, then disrupt ML processes through tampering with the training dataset by somehow introducing malicious samples.
This will result in reduced system reliability, as well as compromised confidentiality and availability of the system.
There are a few ways that threat actors may use data poisoning:
- Crafting special input data to evade intrusion detection systems and then reach internal system
- Injecting misleading samples directly into the training data to change the behavior of say, a malware detection system
- Using “crowdturfing” – creating large numbers of user accounts with false data to mislead the ML classifier systems
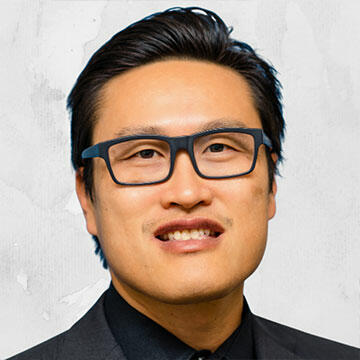
CybersecAsia: What makes data poisoning attacks challenging and time consuming to spot?
IL: Data poisoning attacks can be challenging to detect because only a small amount of poisoned data impacts the entire dataset. The threat actors leverage the many data sources with a massive corpus, which make it almost impossible to validate and curate the data.
At the same time, the explosion of IoT devices has led to massive amounts of data being collected. As data collection becomes more vast and heterogeneous, the features of data are also more complex to understand. This means that attackers have more chances to manipulate the data collected from various sources.
CybersecAsia: How is a data poisoning attack orchestrated? With generative AI taking root, what other repercussions are on the cyber horizon?
IL: Data poisoning can occur once hackers gain access to an AI model’s private training dataset.
Instead of attacking from the outside, thread actors who have gained access to the insides of a system can then attempt to make the inputs accepted into the training data, thereby affecting the system’s ability to produce accurate predictions.
In the ongoing cybersecurity war, threat actors have taken note of the increasing use of AI and ML in detection and protection software to predict and preempt malware activity through data analytics. Hence, using data poisoning can circumvent this detection system and breach the AI/ML cyber threat defenses.
Other repercussions of data poisoning emerging:
- Our research has indicated that vulnerability exploitation shows no sign of slowing down (up from 147,000 attempts in 2021 to 228,000 in 2022). Threat actors are exploiting vulnerabilities that are already disclosed and also ones that are not yet disclosed. Since threat actors are always looking for more advanced techniques to evade security detection and detect vulnerable systems to infiltrate, the use of data poisoning is set to unleash new sophisticated ways of exploiting known critical vulnerabilities and zero day bugs.
- As a result, organisations that are already stretched to the limits in guarding against cyberattacks will soon add another level of cyber vigilance: to simultaneously guard against new, sophisticated attacks as well as attacks built to exploit old vulnerabilities. In this constant race between attackers and defenders, security practitioners are under pressure to find new ways to keep up with cyber threats despite the use of — and now because of the use of — AI and ML.
CybersecAsia: What are the immediate and/or unique cyber measures that can be taken to detect the various types of data poisoning?
IL: Some countermeasures against data poisoning attacks:
- Training data filtering – A data sanitization tactic can be used to separate and remove malicious samples from normal ones. This is done through detecting changes of feature characteristics of training data, or identifying as well as removing data outliers suspected to be malicious.
- De-Pois approach – This is an attack-agnostic approach in which a mimic model imitating the target model behaviour is constructed. This will allow straightforward identification of poisoned samples from the clean ones — through comparisons of prediction differences.
- Developing AI models to regularly check for anomalous data — This can help ensure that all the labels in the training data are accurate and normal.
- Pentesting – using simulated cyberattacks to expose gaps and liabilities that allow threat actors into the system in the first place, before data can be poisoned.
- Adding a second layer of AI and ML — This layer can be tuned to catch potential errors (poisoned or otherwise) in the training dataset.
In addition, organizations can also implement standard best practices such as Zero Trust measures to protect the integrity of the AI/ML environments. This approach assumes that the AI/ML environment is under threat, and every layer of the organization has to be protected by defense-in-depth measures.
Zero Trust principles also advocate against implied trust by enforcing deep inspection and continuous validation on all digital interactions (users and machines).
Finally, Zero Trust means having the ability to quickly respond to cyberattacks by leveraging automation and continually looking out for sophisticated attacks.
CybersecAsia thanks Ian for sharing his insights on data poisoning.