While criminals weaponize AI, banking SOCs are harnessing AI and machine learning capabilities to offer a transformative countermeasure.
Cybercriminals are weaponizing AI in attacks towards the banking sector. The ability for traditional security measures to hold their ground is being threatened at the expense of the vast troves of sensitive data in banks that house a wealth of personal information and account details.
The level of complexity increases when we look at how modern banks now run their businesses across a mix of online platforms, ATMs, and interconnected networks (many of which are cloud-based) creating a wider attack surface.
To shed light on the critical new role AI and LLMs play in defending banks and financial service providers, CybersecAsia sought out insights from Jake King, Head of Threat and Security Intelligence, Elastic.
How are AI threats evolving, especially in the banking and financial services sector?
Jake King (JK): Modern banks operating in the age of AI are experiencing a new wave of security challenges. The rapid evolution of automation, adversarial research, and our collective ability to leverage AI create a cyber threat landscape that continues to pose a challenge for all industries, making it significantly challenging for many organizations to detect and respond to attacks effectively.
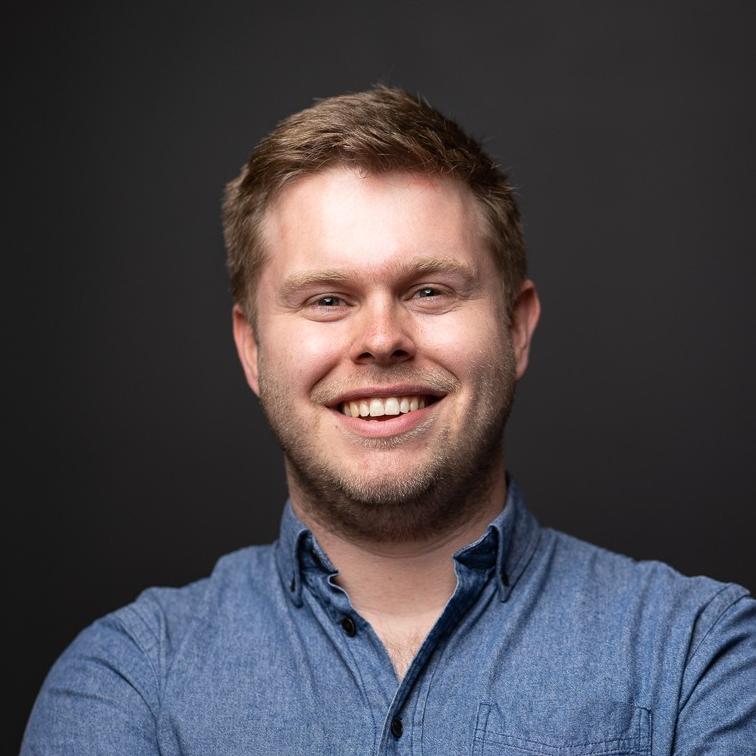
How are AI threats evolving, especially in the banking and financial services sector?
Jake King (JK): Modern banks operating in the age of AI are experiencing a new wave of security challenges. The rapid evolution of automation, adversarial research, and our collective ability to leverage AI create a cyber threat landscape that continues to pose a challenge for all industries, making it significantly challenging for many organizations to detect and respond to attacks effectively.
AI and machine learning allow organizations to analyze, process and automate actions for vast amounts of data, as Large Language Models (LLMs) help streamline workflows and enhance efficiencies. However, the same technological advancements pose significant security challenges, and this advantage also applies to those with nefarious outcomes in mind.
While we observed traditional phishing emails and scams in the early days of AI tool usage, this remains a concern even today as the landscape of cyberthreats has evolved beyond social engineering. AI tools are being leveraged to construct malware, explain complex operations to adversaries and act in ways that lower the technical barrier to a once-sophisticated attack.
Generative AI-powered chatbots can be weaponized without proper security through data poisoning and manipulation. Data poisoning involves feeding bad data into an AI model to make it produce inaccurate or misleading results, while data manipulation involves tricking an AI model into giving away confidential information or behaving in a way it was not designed to. AI-powered bots can also automate such data poisoning and manipulation. It is imperative to implement appropriate controls, and monitor for changes to the software, model, and tools leveraged as part of your AI journey.
As AI becomes more complex, so will the tactics used to exploit it. Integrating AI into the banking sector’s cybersecurity framework encapsulates the technology’s dual nature as both a potential risk factor and a critical defensive tool. By embracing an integrated approach that emphasizes security by design, ethical development practices, and collaborative innovation, banks can harness AI’s full potential to fortify their cyber defenses.
Can LLMs offer a countermeasure to these threats?
JK: There are significant parallels between LLM security and traditional detection engineering. Many security companies are now integrating AI into their security frameworks, and AI for security is one of those first use cases, with LLMs being a core component of this technology and accelerating our ability to defend.
While LLMs cannot directly patch vulnerabilities in AI systems, they can analyse vast amounts of security data from transaction logs, network activity, and customer interactions to detect patterns and help banks investigate complex attacks more efficiently. At Elastic, we’ve opted to leverage LLMs to detect and report malicious activity in environments by examining the stages of an attack and reconstructing these events into a timeline, allowing for faster and more effective remediation.
Leveraging LLMs does not need to stop at processing and automated response, as they can be leveraged to augment existing skills around the authorship of detection rules, response guide development and risk classification. Security teams rely on detection rules to identify threats, and leveraging LLMs can improve the complexities normally associated with rule development and optimization.
Where LLMs are used to deliver consumer services, FSIs must employ integrated measures to counter novel security threats effectively, such as incorporating context-aware prompt filtering and response analysis systems. These systems are designed to understand the broader context of user inputs and responses from the LLM, enabling them to detect and block subtle manipulation attempts more effectively.
This deeper understanding helps distinguish between harmless and potentially harmful inputs, safeguarding the LLM from exploitation – many vendors implement these controls using LLMs and other ML detection mechanisms.
Can LLMs be trained on specific FSI security datasets to improve their accuracy in identifying financial fraud, insider threats, or money laundering activities? How?