How to maintain the fine balance between strong cybersecurity and good customer experience.
Accelerated digital adoption and fast-growing e-commerce amid an extended global pandemic have given rise to a sharp increase in scam and identity fraud cases.
In South-east Asia alone, 70 million people have become digital consumers since the beginning of the pandemic. As more people embrace e-commerce, digital payments have surged, creating more opportunities for cyber criminals to commit fraud.
According to Malin Holmberg, CEO, EMEA & APAC, Experian, 2020 saw an estimated US$27bn in e-commerce transaction fraud, and projections indicate this figure may exceed US$52bn by 2025.
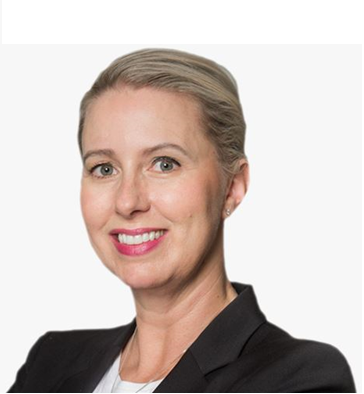
Globally, concerns around fraud and security remain high amongst consumers, with top concerns being stolen credit card information (33%), online privacy (32%), identity theft (32%), and fake/phishing scams (31%).
Regionally, the data shows that consumers in Japan (89%), Australia (83%) and Singapore (82%) consider security to be the most important factor when it comes to their digital experience. In fact, consumers surveyed in India (79%) and Singapore (70%) expressed the highest need to know why their data is being requested: much higher numbers than before the pandemic.
So, what can we do about the alarming trends around heightened sensitivity over data privacy amid digital fraud trends and the business imperative to reduce customer friction? Holmberg provides some answers:
How can businesses balance stronger fraud risk prevention strategies with maximizing customer experience?
Malin Holmberg (MH): Our internal data shows a shift in digital expectations: more than two-thirds of APAC consumers will abandon a transaction if they have to wait more than 30 seconds.
This means fraud checks and authentication measures need to be fast and seamless to improve loyalty and trust. However, all efforts towards building brand loyalty will be ineffective if businesses fail to differentiate true customers from fraudsters and bots.
The answer is to adopt targeted verification strategies using data, software and advanced analytics. By leveraging data from existing and alternative data sources, businesses can facilitate accurate recognition and authentication of customers on a continuous basis.
The latest anti-fraud software adopt biometric recognition of users, thereby enhancing fraud detection further. The same insights that companies use to improve customer experience can power continuous authentication and reduce friction across the customer journey.
Is there a one-size-fits-all anti-fraud solution? If not, how does an organization or industry find the best fit for its needs?
MH: Existing customer data generates a large amount of actionable insights that can help businesses make better decisions against fraud cases. Additionally, AI and ML can effectively trawl through large data sets to identify potential fraud cases.
By combining the three elements (data, analytics and software), businesses can significantly improve the process of fraud detection, resulting in better business outcomes at every stage of the customer lifecycle.
Regarding the general approach to anti-fraud measures, the key elements to address are data, advanced analytics and software that preserve positive customer outcomes and experience amid the detection and intervention of fraud and identity authentication.
One important aspect of a holistic anti-fraud solution is to prioritize investments in technology. Globally, the adoption of AI and machine learning has significantly increased. AI is up 74% from 69% in 2020, and machine learning is up 73%, from 68% in the same period. India is currently leading, with most businesses implementing AI (87%) and machine learning solutions (83%).
How often do advanced analytics and other fraud detection/prevention technologies make an error or contain algorithmic bias? Are there safeguards against this?
MH: ‘False positives’ are common in fraud detection systems and there are a number of ways to address them.
Being reliant on a single system will increase error rates, so I advocate an approach that uses a suite of fraud detection capabilities, orchestrated and combined into a single, higher probability outcome.
For instance, an anti-fraud platform can combine data drive ID checks with passive and active biometric checks for an algorithmic model to analyze. The more reference points from different fraud detection services, the less likely a false positive will result.
Secondly, the feedback loop is very important. When false positives are detected, that data must be fed back into the system. By training the system with proven fraud and false positive data, both false positives and fraud detection rates will improve.
For machine learning implementations, businesses need to tread carefully with unsupervised models. In most cases, a supervised model is recommended to reduce the risk of errors being exaggerated, through the right checks and balances.
How does an organization actually know how many attempted fraud or scam attempts it is thwarting with a newly adopted tech or software, compared to previously?
MH: Any suspected fraud case is normally pushed into a referral queue where an organization’s team of fraud specialists will investigate the case.
Fraud case management software can then support these specialists to examine a suspected case in detail by cross referencing the case with other potential cases, using visual techniques to map out potential fraud rings and fraud schemes.
Once a specialist confirms a fraud case, it will be tagged accordingly, and this data will then be fed back into the system to improve the understanding of a confirmed fraud case.
Additionally, a collective effort can be helpful in addressing fraud cases. For instance, anti-fraud platforms can offer consumers in a particular segment or industry the option of sharing their transaction data anonymously and securely to enrich the data pool for machine learning.
This collective ‘memory’ of transactions is a very powerful data set that, when shared across a consortium, can benefit new member organizations that would otherwise start with a blank slate of machine learning data.
This interview first appeared in DigiconAsia.