A three-step approach can be used to temper the risks of generative AI while exploiting the technology’s strengths to foil cybercriminals.
The Asia Pacific region is one of the most attacked regions. Further complicating the region’s cybersecurity landscape is the arrival of foundation and Large Language Models (LLMs) in generative AI (GenAI).
While the technology has garnered massive interest worldwide, it is now widely seen as a double-edged sword, causing concern for enterprises worldwide. It is important we recognize that cybercriminals are seeking the same benefits.
How should CISOs rethink their security posture? What are the hidden costs and benefits of generative AI on cybersecurity?
AI models can be attacked
A key vector for the downside of GenAI’s impact is that it has given cyberattackers a whole new arsenal. For instance, many small- and medium-sized businesses that do not have adequate security resources may be likelier to leverage foundation models for quick, accessible security support. With LLMs designed to generate realistic outputs, it can also be quite challenging for unsuspecting users to discern incorrect or malicious information.
In one recent experiment to explore the security risks posed by LLMs, and how persuasive or persistent their impact was on delivering directed/incorrect/potentially risky responses and recommendations — one of the key conclusions was that the English language has essentially become a “programming language” for malware.
With LLMs, attackers no longer need to rely on Go, JavaScript, Python, etc., to create malicious code: they would just be required to understand how to effectively command and prompt an LLM using English.
Also, it was concluded that the attack scale and impact that attackers would be able to achieve by compromising LLMs directly make it a very compelling cyber target. In fact, the return-on-investments from compromising AI models is suggesting that attempts and efforts to attack AI models are already underway.
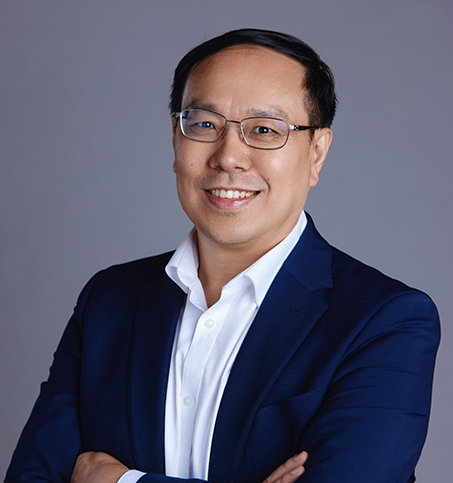
Reversing the negative effects of generative AI
Data is what fuels GenAI — which is why training data has become cyberattack target. The idea is that, if cybercriminals can change the data driving an organization’s generative AI model, they can influence business decisions with targeted manipulation or misinformation.
To prevent expensive and unnecessary consequences, CISOs can adopt a three-step approach:
- First, address data cybersecurity and data provenance issues head-on. It is crucial for CISOs to discover and classify sensitive data used in training or fine-tuning, and implement data loss prevention techniques to prevent data leakage through prompts.
- This is followed by enforcing access policies and controls around ML data sets and expanding threat modeling to cover GenAI-specific threats like data poisoning and outputs containing sensitive data or inappropriate content. When data security is enforced, firms can now use poison to fight poison, i.e., use GenAI to guard against GenAI’s negative impact. When applied to cybersecurity, generative AI can in fact be a business accelerator. Organizations can automate routine tasks that do not require human expertise and judgment, and use GenAI to streamline tasks that rely on the collaboration between humans and technology, such as security policy generation, threat hunting, and incident response. This helps free up teams to focus on the more complex and strategic aspects of security. GenAI can also be used to detect and investigate threats and learn from past incidents to adapt response strategies in real-time, allowing teams to continuously stay one step ahead of new threat vectors.
- The last step to take is to build trust and security in AI use. Organizations need to prioritize data and AI policies and controls centered on security, privacy, governance, and compliance. One way is to tap the open-source community to help draw expertise from across the industry and allowing the community to contribute updates to monitor and improve GenAI continually.
By investing in data protection measures such as data tracking and provenance systems; speeding up security outcomes with GenAI; and embedding elements of trustworthy AI, organizations can improve protection against the technology’s negative impacts.
As more organizations start re-examining their security postures and embrace GenAI intelligently and vigilantly, the region may hopefully become less vulnerable or attractive to threat actors.