As global shortages in cybersecurity talent persist, the use of AI/ML can buffer the heavier workloads — just beware of the pitfalls…
Cybersecurity is a victim of its own success. As more products and services get digitalized, the demand for cybersecurity workers to protect the safety of digital domains has exceeded supply.
Last year, ISC2 estimated that the global shortage of cybersecurity workers has widened by 12.6% to 4m, with those in the Asia Pacific region (APAC) accounting for 23.4%: a shortage of 2.67m cybersecurity workers.
This is also true for identity security, where there are not enough skilled professionals to manage the ever-growing number of identities in enterprises.
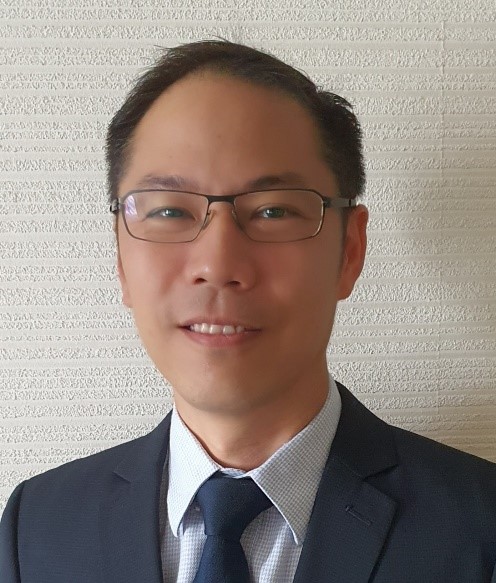
How AI/ML can plug the gaps
The shortage of trained professionals in critical areas like Cloud security and Zero Trust should not be allowed to constrain security teams.
In endpoint security, for example, experienced professionals could be seen spending hours sifting through alerts and creating policies in response. These policies would need to be tested manually before they could be enforced.
However, AI can now be used to make this arduous process significantly easier by prescribing policy recommendations and allowing organizations to confidently set policies, reducing or removing the need for expensive senior analysts’ involvement. This means senior analysts can focus on more pressing tasks.
Although testing outcomes before proceeding to production remains critical, AI can be used to facilitate the overall efficiency of this process. In the meantime, ML algorithms can be used to equip security operations centers (SOCs) to be more nimble in the face of ever more sophisticated threats. ML can help teams analyze large amounts of identity-centric threat data in real-time, and integrate the results with security orchestration, automation and response (SOAR) systems to optimize response workflows, reduce mean time to detection and mean time to response, by reducing the workload on SOC analysts.
Therefore, Generative AI (GenAI) and machine learning (ML) hold immense potential to bolster identity security, particularly in security-policy optimization, risk reduction, and threat detection. Other benefits also include:
- Improving user/threat identification and the understanding of network usage patterns and trends to allow more-informed decision-making, thereby reducing human errors and incidents. For instance, through AI-based ‘user behavioral analytics’ tools, organizations can review large datasets to spot signs of risky user activities and anomalies, something that may be beyond human capabilities because of the large datasets involved. This allows organizations the agility to quickly investigate and address potential issues before they escalate.
- Proactive organizations can also leverage these insights in their educational programs to inform users outside of IT about behavioral patterns to avoid — to help improve security awareness within their organizations.
Not a silver bullet
When adopting new tools into digital architecture, it is critical to ensure they correlate with processes, systems, and policies, especially since the average enterprise IT environment today is complex.
Here is where the human element comes in: although AI and ML can mitigate the skills shortage, it is not a silver bullet. Instead, it should be viewed for what it is: an extraordinary foil to cybersecurity’s key element — people. That rests on contextualizing AI and ML tools to specific use cases in your organization.
Tapping the expertise of external partners to fine-tune, adjust, and streamline will set organizations off to a great start. That can then be a platform to establish feedback loops with internal security teams to drive continuous improvements.
In the final analysis, this is what will arm organizations with the resilience to ride the digitalization wave in search of accelerated growth.