Cybercriminals have pulled off high-profile stunts to bypass biometric authentication. Can they outwit continually evolving behavioral biometrics solutions too?
In the wake of increasing demand for more advanced and secure digital payments solutions, behavioral biometric authentication is slowly becoming essential.
Analyzing unique behavior patterns, such as typing speed and touch gestures, offers a personalized and secure identification process.
Powered by AI and ML, behavioral biometrics can be continuously adapted to distinguishes legitimate users from potential threats, providing an additional layer of security without compromising the user experience.
However, hackers have already shown they can bypass, spoof or foil such security. Sudhindra Magadi, CTO, Wibmo, explained to CybersecAsia how the technology is striving to become even more watertight.
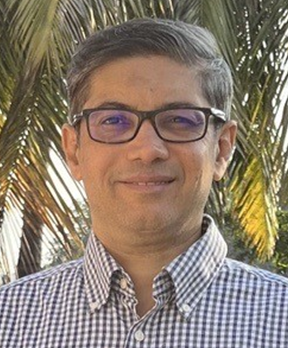
CybersecAsia: What methods or technologies are being used to combat sophisticated spoofing attacks on biometric systems?
Sudhindra Magadi (SM): In the ongoing battle against sophisticated spoofing or impersonation attacks targeting behavioral biometric systems, researchers and developers are exploring various innovative methods and technologies to enhance detection and prevention capabilities. Two notable approaches are continuous authentication and anti-spoofing methodologies.
- Continuous authentication: Instead of just authenticating users during the login, the system can also monitor users during the session to observe their behavior to detect any inconsistencies or sudden changes that resemble spoofing attempts. This real-time monitoring allows immediate intervention, reducing the risk of attacks.
- Anti-spoofing methodology: Device sensors such as gyroscopes and accelerometers are being used to detect any abnormal behavior of users or any synthetic inputs. Also, AI and ML techniques can flag abnormalities by observing the pressure being applied to mouse clicks, keystrokes, or touches.
CybersecAsia: So on top of scanning biometrics, AI and ML are the engine behind behavioral biometrics?
SM: Yes. The integration of AI and ML powers anomaly detection, improved accuracy, and context-based analysis. AI/ML-powered systems can detect deviations from the users’ typical patterns that the systems would have established as baseline behavior. AI and ML can also learn continuously about the user by using a technique called “reinforcement learning”.
With prolonged training, the behavioral biometric models can detect changes in aspects such as typing speed, typing patterns, mouse movements, voice, and touch gestures. This helps reduce false positives and false negatives. Various contextual factors such as device characteristics, geo-location data, time of usage, etc., are also considered:
- Risk-Based Authentication (RBA): This model assesses the risks per transaction by using a combination of factors such as the fingerprint of devices, the user’s behavior, and contextual behavior patterns. When a transaction is deemed risky, additional authentication such as biometric push notifications can be used to validate the user’s identity.
- Sequence-oriented authentication: This involves using many authentication factors, one by one. For example, a user can be authenticated during login via a password, PIN, facial recognition, or fingerprint scanning. Once that is done, when users are making a transaction, they can be prompted to enter specific credit card details. This enhances security by requiring multiple authentications using various factors.
- Mobile specific patterns: On mobile devices users’ specific behavior patterns can be constructed using a combination of factors such as touch gestures, accelerometer or gyroscope metrics, screen interaction patterns, and typing speed. AI and ML models can accurately authenticate the users using pre-learned baselines of these behavioral patterns.
- Mobile device integrations: Behavioral biometric solutions can leverage device-specific features such as Trusted Execution Environments, secure enclaves, or biometric sensors (fingerprint scanners, facial recognition) to enhance the security and accuracy of mobile systems.
Furthermore, to achieve frictionless authentication, employing continuous behavior monitoring as a background activity can reduce the tedium for legitimate users.
CybersecAsia: How are financial institutions and payment service providers managing false positives/negatives in behavioral biometric systems and balancing security with user friction?
SM: They are doing so by implementing various strategies:
- Risk-based thresholds: This technique sets various thresholds based on the transaction type. Low-risk transactions would have lenient thresholds, whereas higher-value ones would have stricter ones. For example, if a transaction is worth a few hundred dollars, the level of risk is low, and hence, only certain rules could be applied; whereas if the transaction is from a different country, then multiple authentication methods could be used.
- Deep data collection and continuous analysis: This technique involves gathering a range of diverse behavioral data patterns from a large user base: large data sets aim to minimize false positives. This improves accuracy over a period of time. This data is used to build various data models that evolve, helping to reduce false positives.
- Fallback mechanisms: Supposing the facial recognition does not work due to poor lighting conditions, alternate methods could be implemented, such as PINs, passwords, patterns, or one-time passwords. This way, the user experience is given high importance.
CybersecAsia: How inter-operable are behavioral biometric solutions across payment platforms?
SM: Some prominent ongoing industry collaborations aimed at standardizing behavioral biometric solutions across different payment platforms are:
- FIDO (Fast Identity Online): An open, scalable, and interoperable authentication standard. FIDO have been driving specifications for password-less authentication methods. They promote standard protocols such as FIDO2 and Webauthn.
- EMVCo: This global body manages the EMV specifications widely used in the payments industry to ensure interoperability and security. Its efforts aim to establish guidelines and provide payment platforms that can seamlessly interact.