The criticality of data resonated in discussions around World Backup Day. While cyber resilience helps ensure the quality and quantity of data for AI projects, immutable data is also critical to cyber resilience.
With World Backup Day recently commemorated, much of the focus has been on the proliferation of data needed to fuel the current AI frenzy.
But for data to be truly useful, organizations need such data to be available and accurate, especially if AI projects are core to business needs. AI is only as good as the available data – it’s a ‘garbage in, garbage out’ world out there. Thus the need for cyber resilience.
So we come full circle, since immutable data is key to cyber resilience too.
CybersecAsia discussed the issues with Sathish Murthy, Senior Systems Engineering Lead, Cohesity ASEAN & India:
How does backup data help ensure cyber resilience?
SM: Cybercriminals can demand ransom payments, often multiple times, from businesses affected by cyberattacks, as these businesses are keen to resume operations as soon as possible. The longer these organizations remain incapacitated, the higher the losses and damage they can suffer in revenue, reputation, and stakeholder trust – making them more likely to pay the ransom to resume operations swiftly.
The rapidly worsening cyberthreat landscape of today means that protecting, managing, securing, and recovering data is harder than ever because cyberattacks and data breaches are now unfortunately a ‘when’ and not ‘if’ reality.
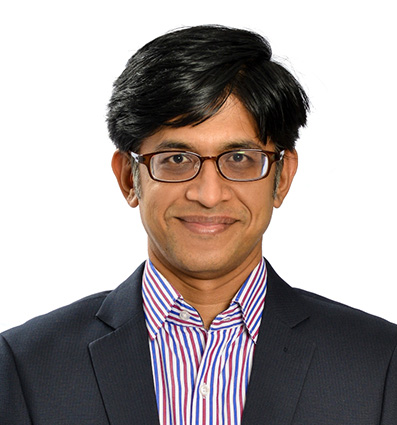
It is vital that organizations have a data backup and recovery strategy that enables them to aggressively recover their data to a predefined point in time (recovery point objective) and within a predefined period (recovery time objective). This is fundamental for cyber resilience – the ability for businesses to sustain operations and revenue streams even after an adverse cyber event.
For one manufacturing company, immutable backup was a saving grace when it fell victim to a ransomware attack. Despite initial projections indicating a prolonged recovery period of two to three weeks, most operations were restored within just three days. The effective use of backup data helped obviate the need for ransom payment and expedited the recovery process by 11 to 18 days, saving the company an estimated USD12 million in potential lost revenue.
How can organizations in Asia Pacific leverage their backup data for different use cases?
SM: Data is an enterprise’s most valuable digital resource. Yet legacy backup point products fragment data across infrastructure silos, management systems, and locations, making it too complex for IT to find, protect, and leverage for business differentiation.
Hyperconverged, software-defined backup infrastructure eliminates unnecessary data copies and addresses mass data fragmentation by consolidating all backed up data and apps on a single web-scale platform creating a unified, global access to all of an organization’s backup data with a simple Google-like search.
This has been particularly beneficial for employees working in siloed environments in different geographies. For example, in one scientific research and pharmaceutical company, scientists were empowered with the ability to search across all the enterprise’s data, whether in files, SharePoint, or One Drive to facilitate their work. With this accessibility, teams can accelerate test/development delivery both on-premises and across clouds through backup data when it is easily and instantly available.
How can organizations in the region ready themselves to capitalize on backup data and AI for competitive advantages?
SM: As artificial intelligence (AI) and machine learning (ML) become increasingly accessible, cybercriminals are empowered to automate and launch more sophisticated cyberattacks, posing significant threats to organizations’ digital security. To counter these evolving threats, organizations must adopt AI and ML technologies themselves to enhance their cyber defenses.
The effectiveness and accuracy of AI models hinge on the quality and quantity of the data they’re trained on. Biased, incomplete, or inaccurate data can lead to erroneous or biased results, undermining the validity and power of AI-driven insights. Moreover, AI models require continuous access to new data to enhance their learning and refine their accuracy over time.
Organizations that prioritize the collection, storage, and analysis of high-quality data are better positioned to harness AI’s potential and gain a competitive advantage. Yet, in today’s complex, distributed architecture, organizations face challenges in managing their data across and throughout their diverse data estates, which often consist of hybrid or multi-cloud data stores. Therefore, organizations should prioritize:
- Data Aggregation and Unification: Organizations need to simply the process of gathering and consolidating data from all sources to establish a unified data repository that includes all their data stored on-premises, cloud, and at the edge. By dismantling data silos and offering a holistic view of organizational data, this supports the AI tools in detecting patterns, trends, and anomalies. Using backup data that is indexed and aggregated allows organization to leverage AI in a highly effective manner that doesn’t consume too much storage space or compute power on production systems and doesn’t risk direct exposure of their production systems to AI applications.
- Data Optimization: Enterprises are storing more data every day and in many cases this is because of data duplicates. These redundancies can occupy large amounts of storage that require huge investments – not just on the storage side, but also in the overall IT infrastructure such as networking, archival, cloud bandwidth, and manageability. When businesses organize their data sets into more compact structures, this creates a more robust AI tool that can identify trends and patterns more efficiently. With infrastructure spanning private data centers, clouds, and edge locations, organizations that invest in collecting, storing, and analyzing high-quality data will be better positioned to leverage the power of AI.
What specific AI use cases can we expect for enterprises this year and in the coming year?
SM: As businesses navigate the trend towards adopting AI and other emerging technologies, it’s crucial to select digital tools that effectively address specific pain points. By leveraging data analytics and insights on data backups, organizations can pinpoint critical areas within their business model that require tailored solutions to support their digital transformation strategies.
This approach ensures that AI adoption is strategic and aligned with overarching business objectives, maximizing the value derived from technological investments.
Enterprises stand to gain significantly from adopting cutting-edge data security, management, and recovery technologies, particularly with the integration of AI and ML in capabilities such as:
- AI-Enabled Multifactor Access (MFA): MFA adds an extra layer of security to ensure that only authorized users access sensitive information. By integrating AI into MFA, organizations can enhance authentication mechanisms based on data risk levels and implement fraud detection systems that automatically block users displaying abnormal access behavior.
- AI Retirement of Inactive Data: Leveraging AI, organizations can identify dormant data suitable for archiving, streamlining recovery processes by eliminating unnecessary retrieval of unused data. This approach not only enhances efficiency but also reduces storage costs.
- AI & ML-Powered Anomaly Detection: AI AI and ML-powered anomaly detection systems alert IT teams to unplanned or abnormal changes in data’s size or format, which is often indicative of malicious activity. By detecting anomalies early, organizations can mitigate potential threats before they escalate and at a minimum have a ‘clean point’ or indication of how to remediate and respond to a cyber or data security incident.
We are also seeing growing popularity of AI-powered assistants. In today’s data-driven landscape, governance and compliance are paramount for enterprises. However, navigating vast amounts of data to ensure regulatory adherence can be a daunting task. Efficiently identifying and addressing potential violations buried within extensive datasets can be simplified through AI.
For example, if a compliance officer needs to investigate potential regulatory violations related to the leakage of bankruptcy details and employee incentives shared through email, this would require a tedious search process.
However, AI-powered conversational search capabilities enable rapid analysis of data, allowing the compliance officer to swiftly uncover pertinent information. Beyond just pulling up data, AI-powered search engines can point users to other relevant information from a company’s data stack without further prompting.
Developing a highly contextual, domain-specific models represent a significant leap forward in knowledge-grounded conversations. By using the power of an organization’s backup data and incorporating both the topic and local context of a conversation, AI advancements can generate more knowledgeable, diverse, and relevant responses to accelerate the goals of an organization.