More than US$1tn flows through money launderers worldwide despite tightened vigilance and audits. What are the technology dynamics in play?
According to the Financial Accountability Transparency & Integrity panel (FACTI), businesses lose around US$1.6tn per year due to money laundering activities worldwide.
In the wake of recent spectacular crackdowns on multi-billion dollar money laundering scandals, people in the region are curious: were the criminals assisted by technology to pull off their crimes undetected for so long? What technology aided the authorities in their capture?
CybersecAsia.net interviewed Mike Foster, President and CEO, SymphonyAI/Sensa-NetReveal, for some insights on the anti-financial crime (AFC) landscape.
CybersecAsia: In the wake of recent spectacular crackdowns on multi-billion dollar money laundering scandals, people in the region are curious: were the criminals assisted by technology to pull off their crimes undetected for so long? What technology aided the authorities in their capture?
Mike Foster (MF): What we can say with certainty is that, in the context of digital banking, technology can support both anti-financial crime activities and the criminals. Behind a digital barrier, criminals can disguise their identity, use mules to move money, hide criminal networks and perpetrate crime at scale using AI.
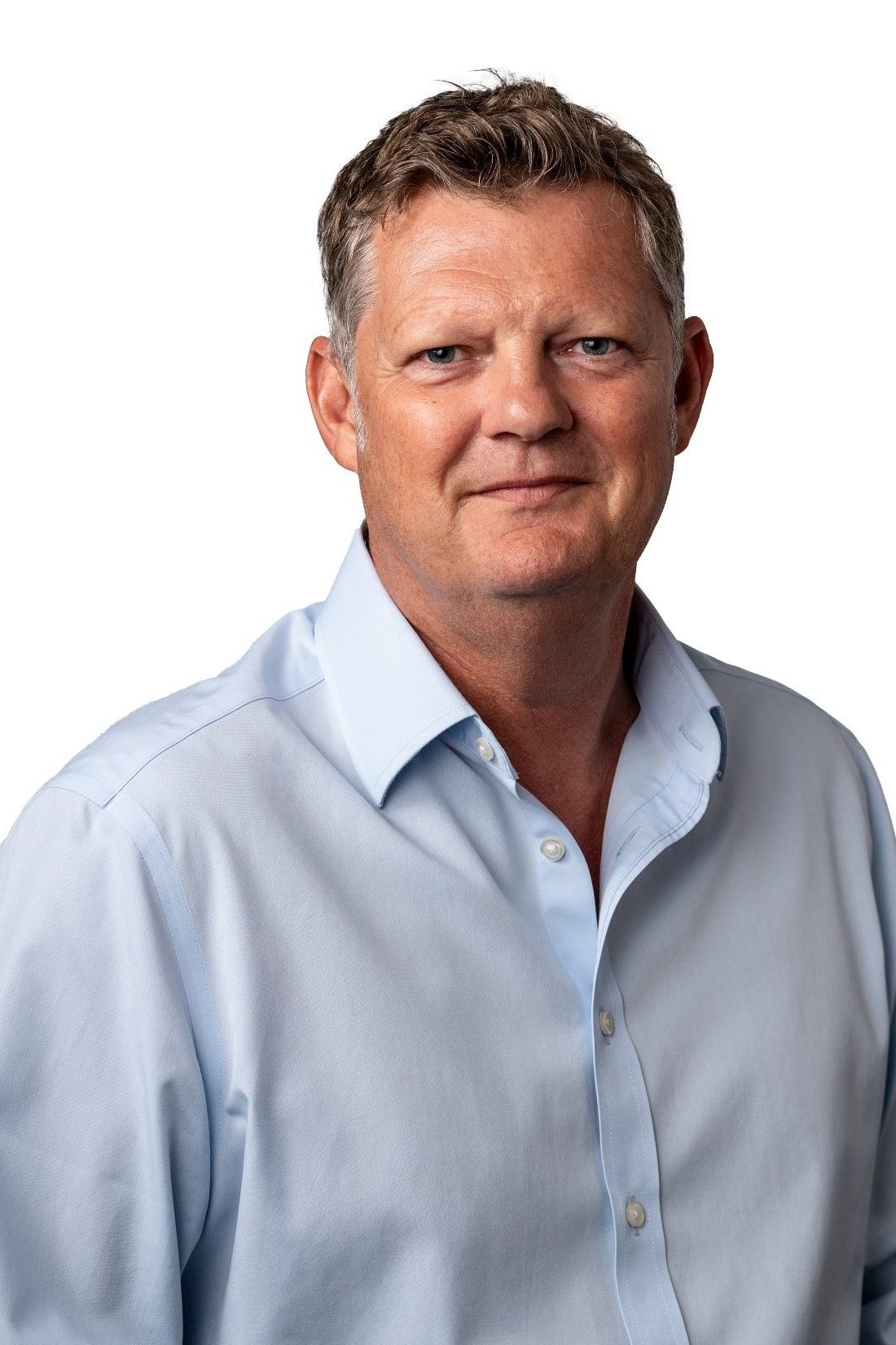
At the same time, anti-financial crime solutions are evolving to keep ahead of criminals. In today’s environment, you are not just trying to find the needle in a haystack; you are trying to find a needle in a stack of a billion needles that all look similar. Leveraging generative AI (GenAI) to fight financial crime is one of the best options for organizations as these intelligent models can help detect suspicious changes in user behavior or run context-aware analyses to help assess risk with increasing accuracy.
Ultimately, tools like generative AI empower investigators to keep up with the volume of alerts and dig deeper into investigations to identify and report suspicious actors.
CybersecAsia: What are the practical use cases of GenAI you alluded to, for use in anti-financial crime (AFC)? How do you see the fight between good AI against bad AI going to turn out in the near future?
MF: AI is only as good (or bad) as the data it is trained on: there is no such thing as “bad AI”. However, there are bad practices that can render an AI model unreliable, inaccurate and biased.
The focus is on responsible AI, and this means AI that is human-centric, secure, robust and unbiased used for supporting critical decisions — not making them. A proactive approach to tackling bias is essential, from continuous monitoring and diverse training data to robust algorithmic fairness techniques.
Practical use cases of GenAI in AFC are abundant. Financial crime investigations are plagued by false positive alerts that slow down/distract investigators and mask genuine risk. GenAI-powered tools supercharge investigations by intelligently aggregating relevant data, providing a natural language narrative that investigators can interrogate and use to summarize draft narratives for reporting purposes. Such an efficient digital assistant takes on the manual tedious work so that human investigators can focus their resources on more critical risks.
Having said that, for all the things that organizations are trying to mobilize and solve, the bad actors are doing the same thing but the opposite. It is a continuous fight. GenAI is modernizing existing anti-money laundering and compliance programs to keep pace with emerging crime methods, knowing that pitfalls will become easier to remedy over time.
CybersecAsia: If I am a fraudster reading this interview, how can I use AI to outsmart the authorities or buy more time to escape?
MF: Predictive and GenAI combined can create powerful tools. For criminals, it has opened opportunities for AI-powered money laundering where they create algorithms to detect and exploit vulnerabilities in transaction monitoring systems, making illicit financial activities harder to trace. They also use machine learning to mimic legitimate transaction patterns, making it more difficult for anomaly detection systems to identify fraudulent activities.
- The selection of datasets for the training and development of models is key, as it influences reliability, accuracy, and susceptibility to bias.
- Intensive testing for bias and hallucinations is another key component of the development process before a GenAI solution is deployed. Developers mitigate these risks with appropriate reinforced machine learning and embeddings.
- Human verification is also required in the testing and validation procedures, so no surprise results or human oversight eventuate. Relevant explainability tools or models should be built into solutions to deliver insights into decision trees or feature importance in relation to a model’s output.