Just as ChatGPT v3.5 was hampered by dated data, GenAI tools can be more powerful if they use the latest data.
The popularity of generative AI (GenAI) technology is set to help organizations in the region leverage AI, automation and analytics.
However, adoption of the technology has led to a twofold challenge: building an adequate infrastructure to effectively harness the potential of AI, while navigating the complexities introduced by GenAI around data validation, governance and security.
How can organizations interact with and manage their data to tackle these obstacles? A key solution to do so is data streaming.
GenAI benefits from real-time data
Many contemporary AI and large language models (LLMs) depend on extensive historical data sets accumulated over many years. Chatbots like ChatGPT would reference data sets that are typically a few months old.
In contrast, data streaming allows data to be processed in a real-time, continuous flow. This allows organizations to power AI models with precise insights extracted from trustworthy data as they are generated, enabling continuous learning and adaptation with the most updated information on hand.
Whether it is a chatbot intelligently identifying the latest transactions when assisting with customer queries, or an advertiser analyzing real-time data to tailor product recommendations for individual consumers, data streaming can fuel GenAI models to offer a whole new level of personalization.
Data streaming platforms also incorporate data governance processes that further help organizations to safely scale, organize and share their data across the business. This provides oversight and insight into who can access, audit and modify the large volume of data streams that are being fed to AI models.
With an additional layer of security and accountability to how data is utilized, organizations can improve the accuracy of their GenAI output while safeguarding data from all their stakeholders.
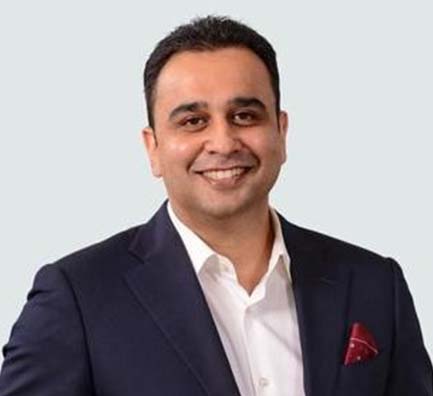
Using AI for cybersecurity
Additionally, the rapid digital transformation in the region has ignited strong growth in cybercrime. Coupled with the increasing sophistication of cyberattacks, the cyber risk landscape requires early threat detection. This is where organizations can tap into the synergy of data streaming and AI to enhance cybersecurity measures.
By instantly connecting all operational and analytical data to create a real-time knowledge repository, organizations can train AI models with ample and up-to-date contextual intelligence, to conduct granular and precise end-to-end threat analytics across their digital channels.
Fraud prevention teams will also be able to run thousands of complex, contextualized rules, map live changes onto a record of customer activity, and automate actions in real time. This will empower firms to rapidly identify anomalies even in periods of peak customer activity, and activate the necessary protocols before irreversible damage is done.
Keeping AI regulations in pace
AI models are only as good as the data they are built on. Yet this is not the only factor when it comes to addressing data security around AI.
Personal, enterprise and national data will need to be used in the right manner. This remains a challenge as regulatory frameworks require thorough discussions and still need to keep up with the speed at which AI is developing. There are also issues around complex and diplomatically sensitive data sovereignty to contend with.
As such, close collaboration between governments and corporations developing the technology is necessary to evaluate and balance the risks, potential for innovation and long-term viability of AI. While regulatory discussions continue, organizations need to re-evaluate their approach to data to maximize the growth that AI has to offer.
By securing and establishing the latest and most trusted data sets as a foundation for their AI models, firms will be well-equipped to leverage AI for their business transformation goals even as the technology and industry continues to evolve.